Catalogue Search | MBRL
نتائج البحث
MBRLSearchResults
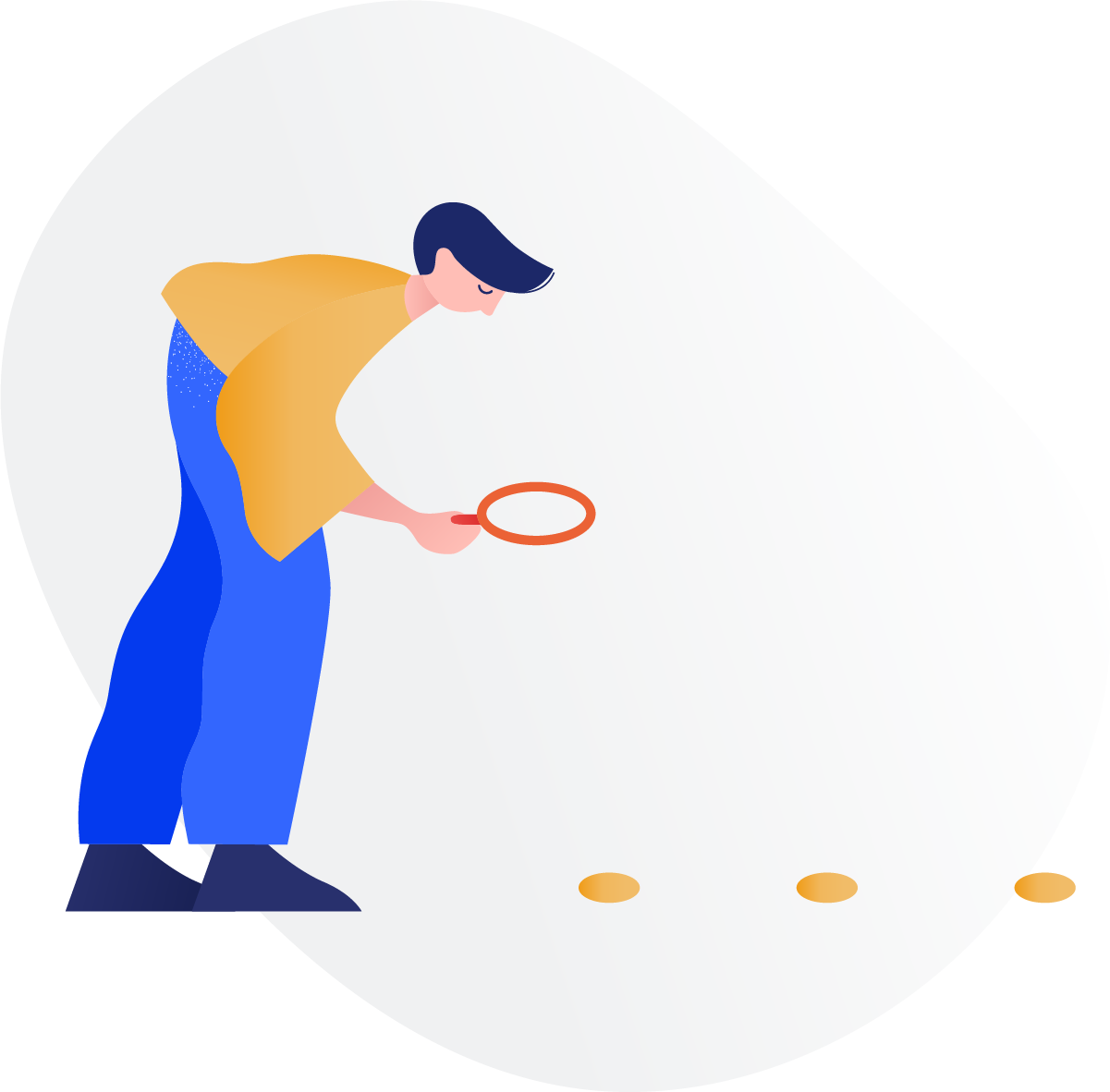
وجه الفتاة! هناك خطأ ما.
أثناء محاولة إضافة العنوان إلى الرف ، حدث خطأ ما :( يرجى إعادة المحاولة لاحقًا!
-
الضبطالضبط
-
مُحَكَّمةمُحَكَّمة
-
السلسلةالسلسلة
-
مستوى القراءةمستوى القراءة
-
السنةمن:-إلى:
-
المزيد من المرشحاتالمزيد من المرشحاتنوع المحتوىنوع العنصرلديه النص الكاملالموضوعالناشرالمصدرالمُهدياللغةمكان النشرالمؤلفينالموقع
منجز
مرشحات
إعادة تعيين
578,971
نتائج ل
"Medical imaging"
صنف حسب:
Image processing and Quality Control for the first 10,000 brain imaging datasets from UK Biobank
بواسطة
Zhang, Hui
,
Miller, Karla L.
,
Hernandez-Fernandez, Moises
في
Alzheimer's disease
,
Automation
,
Big data imaging
2018
UK Biobank is a large-scale prospective epidemiological study with all data accessible to researchers worldwide. It is currently in the process of bringing back 100,000 of the original participants for brain, heart and body MRI, carotid ultrasound and low-dose bone/fat x-ray. The brain imaging component covers 6 modalities (T1, T2 FLAIR, susceptibility weighted MRI, Resting fMRI, Task fMRI and Diffusion MRI). Raw and processed data from the first 10,000 imaged subjects has recently been released for general research access. To help convert this data into useful summary information we have developed an automated processing and QC (Quality Control) pipeline that is available for use by other researchers. In this paper we describe the pipeline in detail, following a brief overview of UK Biobank brain imaging and the acquisition protocol. We also describe several quantitative investigations carried out as part of the development of both the imaging protocol and the processing pipeline.
Journal Article
Mass Spectrometry Imaging and Integration with Other Imaging Modalities for Greater Molecular Understanding of Biological Tissues
بواسطة
Fletcher, John S.
,
Porta Siegel, Tiffany
,
Cappell, Jo
في
allen brain
,
Antibodies
,
ates of america
2018
Over the last two decades, mass spectrometry imaging (MSI) has been increasingly employed to investigate the spatial distribution of a wide variety of molecules in complex biological samples. MSI has demonstrated its potential in numerous applications from drug discovery, disease state evaluation through proteomic and/or metabolomic studies. Significant technological and methodological advancements have addressed natural limitations of the techniques,
i.e.
, increased spatial resolution, increased detection sensitivity especially for large molecules, higher throughput analysis and data management. One of the next major evolutions of MSI is linked to the introduction of imaging mass cytometry (IMC). IMC is a multiplexed method for tissue phenotyping, imaging signalling pathway or cell marker assessment, at sub-cellular resolution (1 μm). It uses MSI to simultaneously detect and quantify up to 30 different antibodies within a tissue section. The combination of MSI with other molecular imaging techniques can also provide highly relevant complementary information to explore new scientific fields. Traditionally, classical histology (especially haematoxylin and eosin–stained sections) is overlaid with molecular profiles obtained by MSI. Thus, MSI-based molecular histology provides a snapshot of a tissue microenvironment and enables the correlation of drugs, metabolites, lipids, peptides or proteins with histological/pathological features or tissue substructures. Recently, many examples combining MSI with other imaging modalities such as fluorescence, confocal Raman spectroscopy and MRI have emerged. For instance, brain pathophysiology has been studied using both MRI and MSI, establishing correlations between in and
ex vivo
molecular imaging techniques. Endogenous metabolite and small peptide modulation were evaluated depending on disease state. Here, we review advanced ‘hot topics’ in MSI development and explore the combination of MSI with established molecular imaging techniques to improve our understanding of biological and pathophysiological processes.
Journal Article
Deep learning in biomedical and health informatics : current applications and possibilities
\"This book provides a proficient guide on the relationship between AI and healthcare and how AI is changing all aspects of the health care industry. It also covers how deep learning will help in diagnosis and prediction of disease spread\"-- Provided by publisher.
A multi-model deep convolutional neural network for automatic hippocampus segmentation and classification in Alzheimer’s disease
بواسطة
Li, Fan
,
Liu, Manhua
,
Xu, Mingqing
في
Aged
,
Aged, 80 and over
,
Alzheimer Disease - diagnostic imaging
2020
Alzheimer’s disease (AD) is a progressive and irreversible brain degenerative disorder. Mild cognitive impairment (MCI) is a clinical precursor of AD. Although some treatments can delay its progression, no effective cures are available for AD. Accurate early-stage diagnosis of AD is vital for the prevention and intervention of the disease progression. Hippocampus is one of the first affected brain regions in AD. To help AD diagnosis, the shape and volume of the hippocampus are often measured using structural magnetic resonance imaging (MRI). However, these features encode limited information and may suffer from segmentation errors. Additionally, the extraction of these features is independent of the classification model, which could result in sub-optimal performance. In this study, we propose a multi-model deep learning framework based on convolutional neural network (CNN) for joint automatic hippocampal segmentation and AD classification using structural MRI data. Firstly, a multi-task deep CNN model is constructed for jointly learning hippocampal segmentation and disease classification. Then, we construct a 3D Densely Connected Convolutional Networks (3D DenseNet) to learn features of the 3D patches extracted based on the hippocampal segmentation results for the classification task. Finally, the learned features from the multi-task CNN and DenseNet models are combined to classify disease status. Our method is evaluated on the baseline T1-weighted structural MRI data collected from 97 AD, 233 MCI, 119 Normal Control (NC) subjects in the Alzheimer’s Disease Neuroimaging Initiative (ADNI) database. The proposed method achieves a dice similarity coefficient of 87.0% for hippocampal segmentation. In addition, the proposed method achieves an accuracy of 88.9% and an AUC (area under the ROC curve) of 92.5% for classifying AD vs. NC subjects, and an accuracy of 76.2% and an AUC of 77.5% for classifying MCI vs. NC subjects. Our empirical study also demonstrates that the proposed multi-model method outperforms the single-model methods and several other competing methods.
Journal Article
Sample size evolution in neuroimaging research: An evaluation of highly-cited studies (1990–2012) and of latest practices (2017–2018) in high-impact journals
بواسطة
Ioannidis, John PA
,
Szucs, Denes
في
Bibliometrics
,
Biomedical Research - standards
,
Biomedical Research - statistics & numerical data
2020
We evaluated 1038 of the most cited structural and functional (fMRI) magnetic resonance brain imaging papers (1161 studies) published during 1990–2012 and 270 papers (300 studies) published in top neuroimaging journals in 2017 and 2018. 96% of highly cited experimental fMRI studies had a single group of participants and these studies had median sample size of 12, highly cited clinical fMRI studies (with patient participants) had median sample size of 14.5, and clinical structural MRI studies had median sample size of 50. The sample size of highly cited experimental fMRI studies increased at a rate of 0.74 participant/year and this rate of increase was commensurate with the median sample sizes of neuroimaging studies published in top neuroimaging journals in 2017 (23 participants) and 2018 (24 participants). Only 4 of 131 papers in 2017 and 5 of 142 papers in 2018 had pre-study power calculations, most for single t-tests and correlations. Only 14% of highly cited papers reported the number of excluded participants whereas 49% of papers with their own data in 2017 and 2018 reported excluded participants. Publishers and funders should require pre-study power calculations necessitating the specification of effect sizes. The field should agree on universally required reporting standards. Reporting formats should be standardized so that crucial study parameters could be identified unequivocally.
•The most highly cited 1000+ neuroimaging studies had sample size of 12.•A sample of about 300 studies published during 2017 and 2018 had sample size of 23-24.•Sample sizes increase at a rate of ~0.74 participant/year.•Only 3% of recent papers had power calculations, mostly for t-tests and correlations.•Design parameters and power calculations should be reported more clearly.
Journal Article
EANM procedural guidelines for radionuclide myocardial perfusion imaging with SPECT and SPECT/CT: 2015 revision
بواسطة
Slart, Riemer H. J. A.
,
Buechel, Ronny R.
,
Acampa, Wanda
في
Adult
,
Cardiology
,
Clinical Medicine
2015
Since the publication of the European Association of Nuclear Medicine (EANM) procedural guidelines for radionuclide myocardial perfusion imaging (MPI) in 2005, many small and some larger steps of progress have been made, improving MPI procedures. In this paper, the major changes from the updated 2015 procedural guidelines are highlighted, focusing on the important changes related to new instrumentation with improved image information and the possibility to reduce radiation exposure, which is further discussed in relation to the recent developments of new International Commission on Radiological Protection (ICRP) models. Introduction of the selective coronary vasodilator regadenoson and the use of coronary CT-contrast agents for hybrid imaging with SPECT/CT angiography are other important areas for nuclear cardiology that were not included in the previous guidelines. A large number of minor changes have been described in more detail in the fully revised version available at the EANM home page:
http://eanm.org/publications/guidelines/2015_07_EANM_FINAL_myocardial_perfusion_guideline.pdf
.
Journal Article